Machine Learning for Predictive Security in Digital Wallet Systems
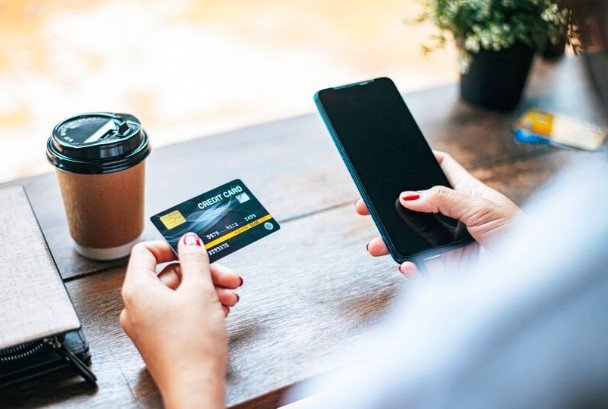
In an era where digital transactions dominate financial activities, the security of digital wallet systems has become a paramount concern. With the rise of cryptocurrencies and the increasing adoption of digital wallets, securing these platforms is essential to protect users’ funds and personal information. Machine learning (ML) is playing a crucial role in enhancing the security measures of these systems, particularly in the context of enterprise crypto wallets. This article delves into how machine learning can bolster predictive security in digital wallet applications, ensuring a safer experience for users.
The Importance of Security in Digital Wallets
Digital wallets have revolutionized the way people manage their finances. From simple transactions to complex enterprise crypto wallet solutions, these platforms provide users with convenience and flexibility. However, the rapid growth of digital wallets has also attracted malicious actors looking to exploit vulnerabilities. Security breaches can lead to financial losses, identity theft, and erosion of user trust. As a result, implementing robust security measures is vital for the sustainability and growth of digital wallet services.
Machine Learning: A Game Changer for Security
Machine learning, a subset of artificial intelligence, enables systems to learn from data and improve their performance over time without explicit programming. This capability is particularly beneficial for identifying patterns and anomalies in user behavior, making it an invaluable tool for predictive security. By analyzing vast amounts of data, machine learning algorithms can detect potential threats in real time, enhancing the security posture of digital wallet systems.
1. Anomaly Detection
One of the most significant applications of machine learning in digital wallet security is anomaly detection. By establishing a baseline of normal user behavior, machine learning models can identify deviations that may indicate fraudulent activities. For example, if a user typically makes small transactions and suddenly attempts a large transfer, the system can flag this behavior as suspicious. This proactive approach helps in preventing unauthorized transactions, especially in enterprise crypto wallets, where the stakes are often higher.
2. Risk Assessment
Machine learning algorithms can assess the risk associated with different transactions by evaluating multiple factors, such as transaction amount, frequency, and user location. This risk assessment can help digital wallet providers determine which transactions require additional scrutiny or verification. By implementing such predictive measures, companies can minimize the chances of fraud and protect users’ assets in their enterprise crypto wallets.
3. User Authentication
Traditional authentication methods, such as passwords and PINs, can be vulnerable to breaches. Machine learning can enhance user authentication processes through biometric recognition, such as fingerprint or facial recognition, and behavioral biometrics, which analyze patterns in user interactions with the app. By employing these advanced methods, digital wallet systems can ensure that only authorized users gain access to sensitive information and transaction capabilities.
4. Continuous Learning and Adaptation
One of the key advantages of machine learning is its ability to adapt over time. As cyber threats evolve, so too must the defenses protecting digital wallets. Machine learning models can be continuously updated with new data, allowing them to learn from emerging threats and adjust their detection algorithms accordingly. This adaptability is essential for maintaining the security of enterprise crypto wallets, which are often targeted due to their high value.
5. Enhanced Fraud Prevention
By leveraging machine learning, digital wallet providers can enhance their fraud prevention strategies. These algorithms can analyze historical data to identify patterns associated with fraudulent behavior, such as the use of stolen credentials or unusual transaction methods. By implementing real-time monitoring and alerts based on these patterns, companies can significantly reduce the risk of fraud and protect their users’ funds.
Conclusion
As digital wallets continue to gain traction in the financial ecosystem, the need for robust security measures is more critical than ever. Machine learning offers a transformative approach to predictive security in digital wallet systems, providing tools for anomaly detection, risk assessment, user authentication, continuous learning, and fraud prevention. For enterprise crypto wallet providers, these capabilities are essential for safeguarding users’ assets and maintaining their trust.
Incorporating machine learning into security protocols not only enhances the protection of digital wallets but also enables companies to stay ahead of evolving threats. As the digital finance landscape continues to evolve, embracing machine learning will be pivotal in ensuring the security and reliability of digital wallet systems, ultimately fostering a safer environment for all users.